Overall approach
The AI4Lab2Plant research project, by the FH OÖ Research Group HEAL (FHOOE-HEAL) in collaboration with K1-MET GmbH (K1-MET) and voestalpine Stahl GmbH (VAS), seeks to advance the practical applicability of Machine Learning in industrial settings. By developing innovative algorithms and integrating domain-specific knowledge, the project aims to create predictive models that are not only effective in controlled laboratory environments but also scalable to large-scale production facilities. This initiative is designed to enhance efficiency, improve product quality, and promote resource conservation across the industrial landscape. Key focus areas of the project include:
- Trustworthiness and Interpretability: The project emphasizes the development of ML models that are transparent and interpretable by domain experts, as such models are generally more trusted than opaque, “black-box” alternatives.
- Knowledge Integration: In data-scarce scenarios, models that can incorporate existing domain knowledge are particularly advantageous.
- Transferability: A significant challenge is ensuring that models developed in a training environment can be effectively transferred to operational industrial settings.
While current ML methods often address these aspects individually, they rarely do so in an integrated manner. The need for a holistic approach is especially evident in the case of voestalpine Stahl GmbH. The project includes functional tests on synthetic data and focuses on the coating process of large-scale components in the steel industry. The goal is to model specific coating properties based on collected data, enabling real-time prediction and optimization in the future. A key feature of the project is the use of symbolic regression to incorporate domain knowledge into the modeling process, thereby ensuring high interpretability of the results.
One of the major challenges is that certain properties can only be observed under laboratory conditions, and their behavior in large-scale industrial settings is often uncertain. To address this, the project plans to combine the models with transfer learning techniques. This will allow for the transferability of prediction models – initially based on lab data and enhanced by domain knowledge – to industrial scales. Successful implementation will improve the coating process, increase the quality of the coatings, reduce maintenance effort for plants such as continuous casting or galvanizing lines, increase predictability and ultimately conserve resources. Additionally, the optimized coating process is expected to reduce the failure rate of industrial components, thereby boosting overall efficiency.
The innovative combination of interpretable, knowledge-integrating, and transferable ML methods proposed in this project has the potential to address similar challenges in other industrial sectors where data may be limited, but domain knowledge is abundant. The project also plans to release the developed methods as open-source software, providing easy access for future users and enabling broader application in other technically driven fields.
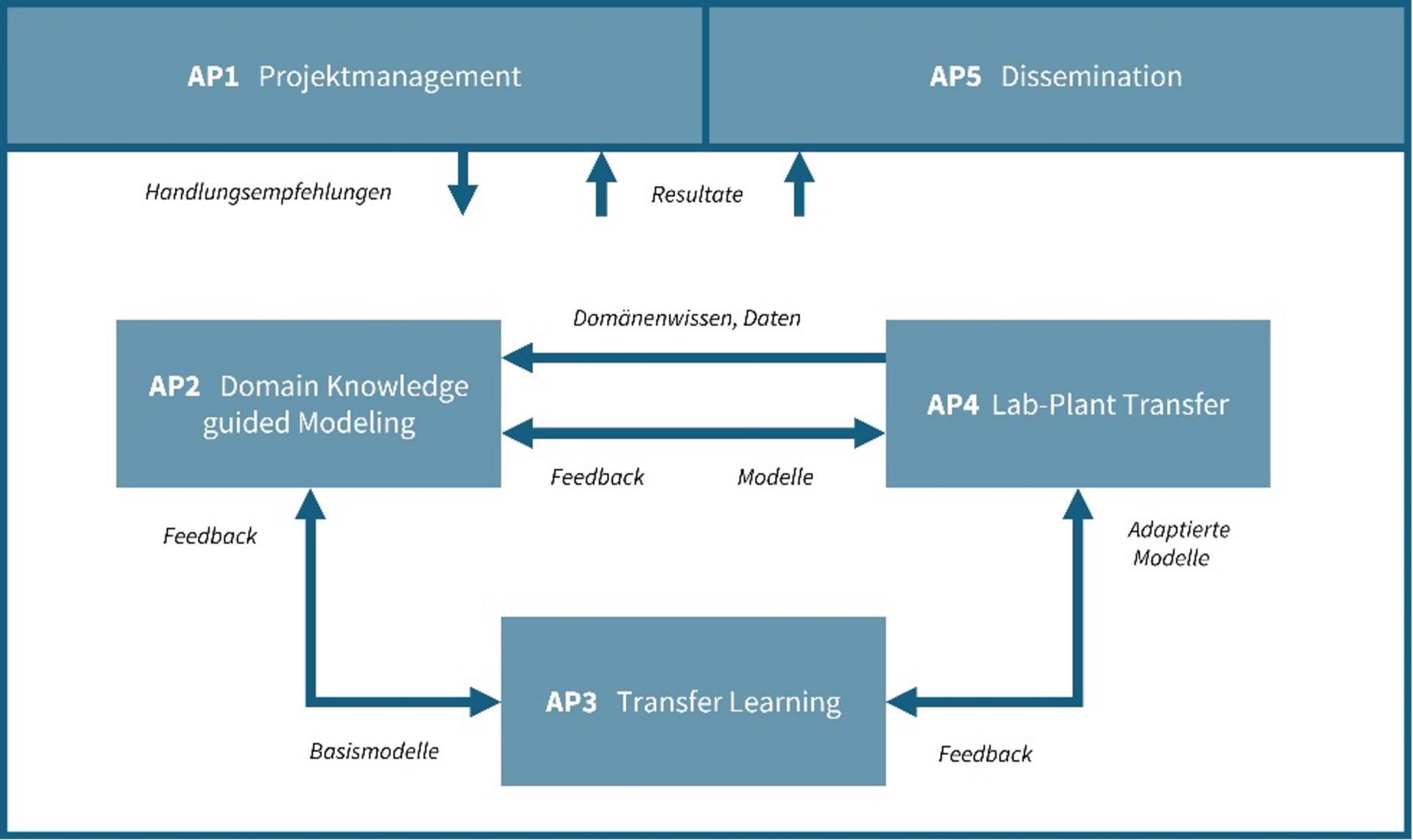
Objectives
The main goal of this project, over its 36 month duration, are is the Artificial Intelligence driven Knowledge Transfer from Lab to Industrial Plant.
PROJECT TIMELINE:
1 January 2025 – 31 December 2027
FUNDING SCHEME:
AI4Lab2Plant is a cooperative R&D project of FH OÖ Forschungs- und Entwicklungs GmbH, K1-MET GmbH and voestalpine Stahl GmbH, funded by the AI Region Upper Austria initiative of the state of Upper Austria as part of the economic and research strategy #upperVISION2030.
PROJECT CONSORTIUM:
Starting with the project coordinator, the consortium is composed as follows: